Thought Leadership
Hear from thought leaders across the Planhat community.
Thought Leadership
Hear from thought leaders across the Planhat community.
Thought Leadership
Hear from thought leaders across the Planhat community.
Thought Leadership
Hear from thought leaders across the Planhat community.
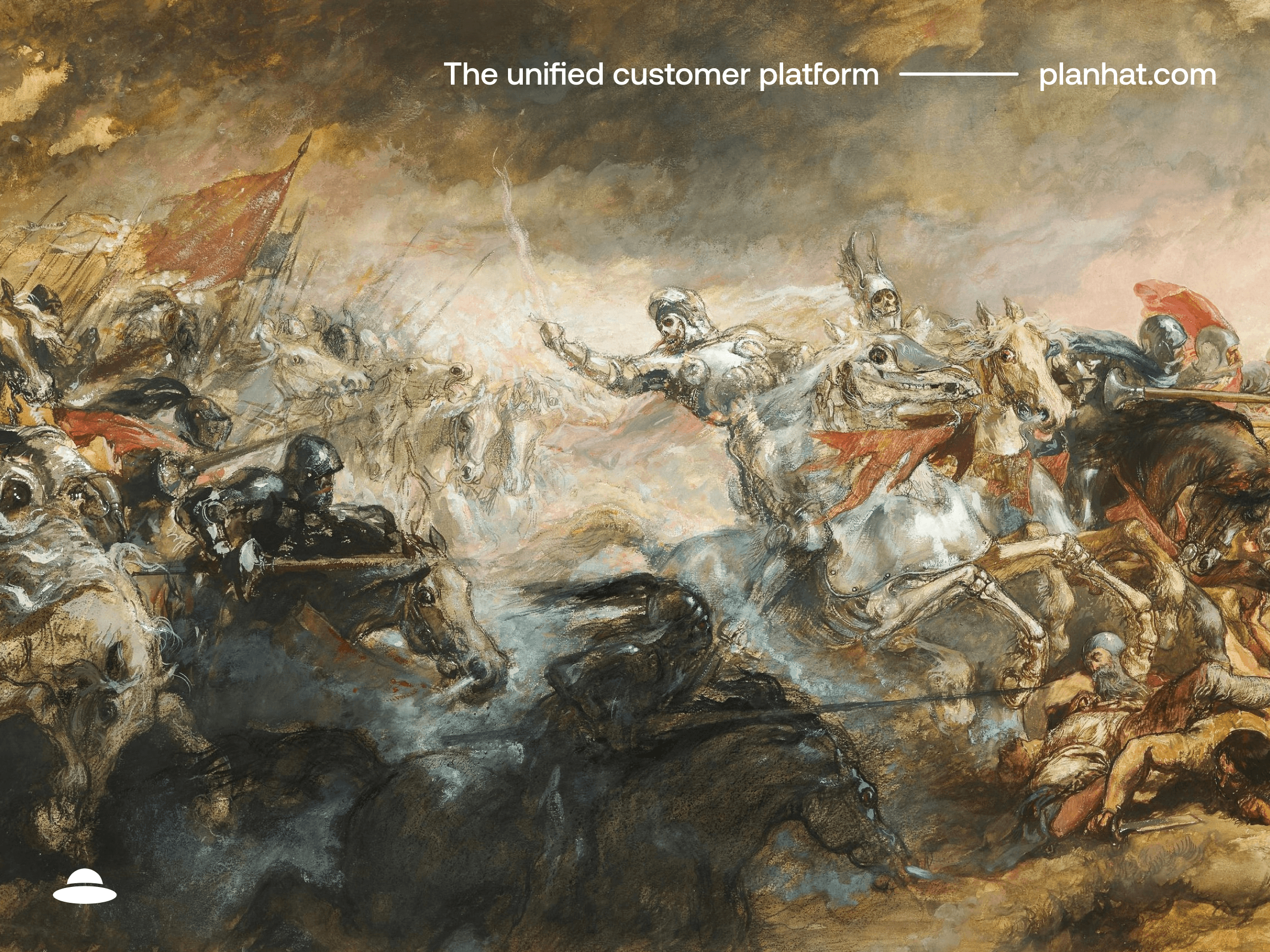
Spotlight
Perspective
•
5 minute read
The infinite frontier
Discover Planhat's philosophy on Security, centered around dynamic protocols rather than static protections.
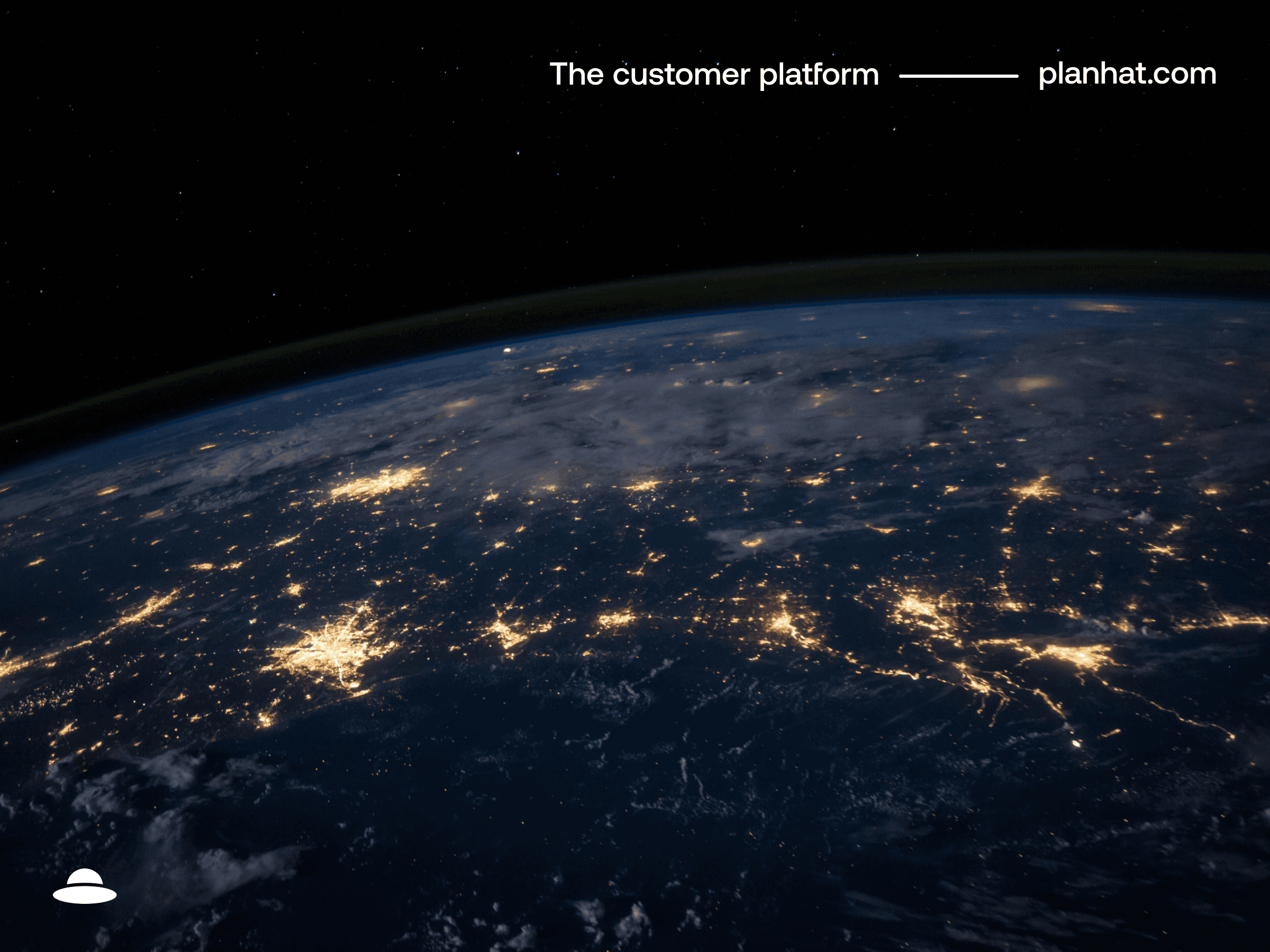
Spotlight
Vendor Assessment
•
10 minute read
Planhat is named a Customer Success Leader by IDC
Planhat is a Leader in IDC's 2024 MarketScape across both current capabilities and market-readiness of future strategies.
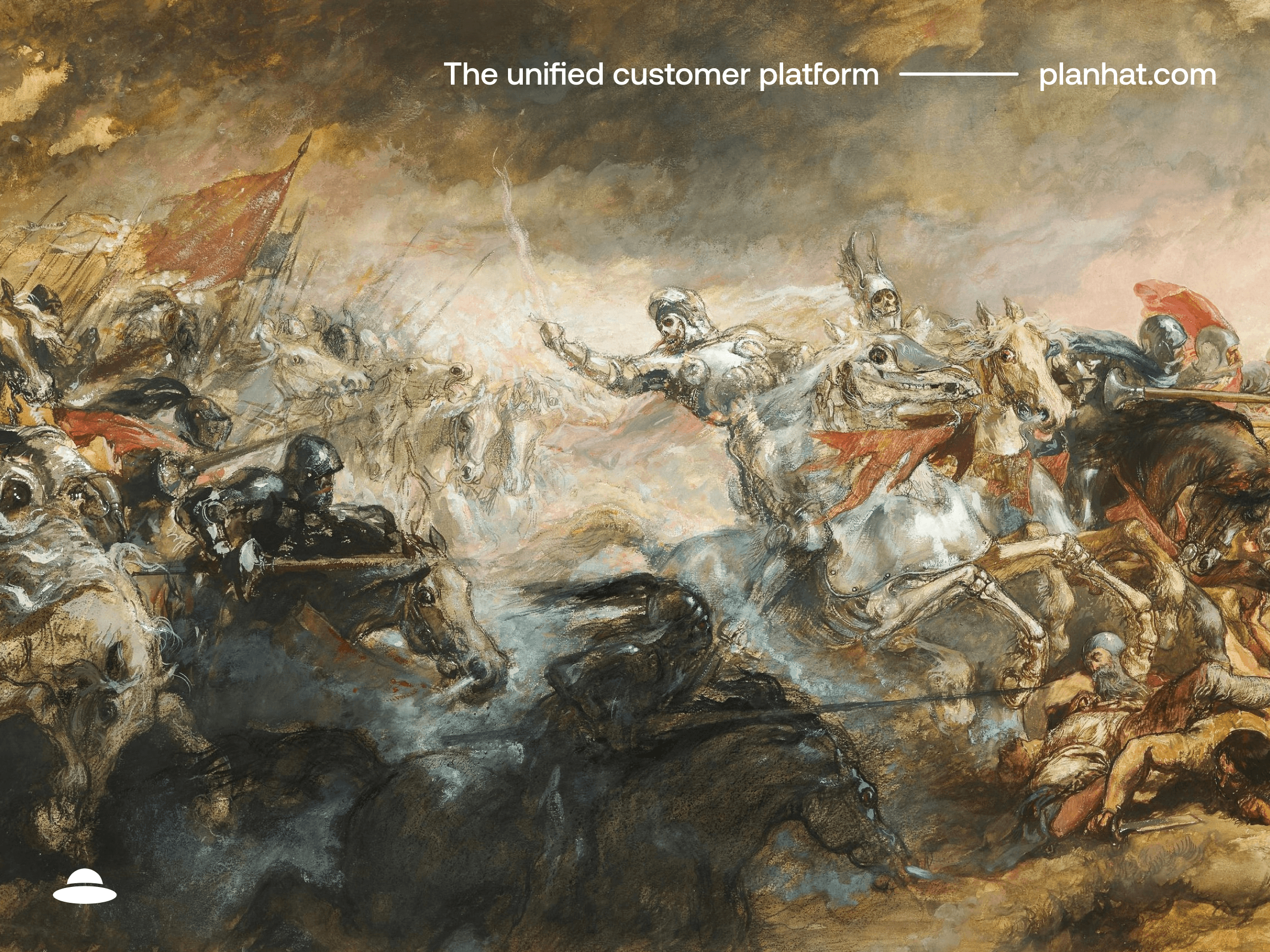
Spotlight
Perspective
•
5 minute read
The infinite frontier
Discover Planhat's philosophy on Security, centered around dynamic protocols rather than static protections.
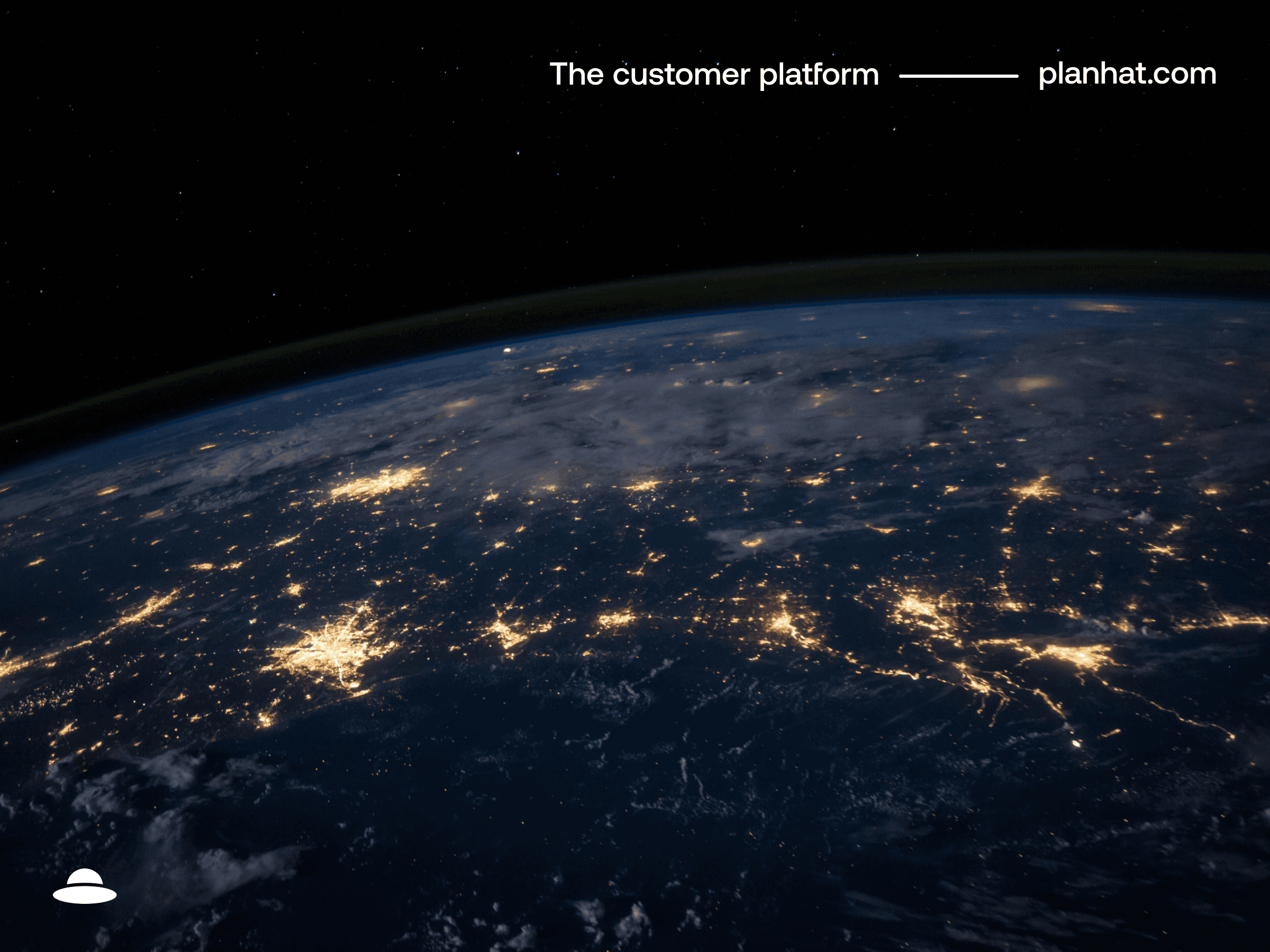
Spotlight
Vendor Assessment
•
10 minute read
Planhat is named a Customer Success Leader by IDC
Planhat is a Leader in IDC's 2024 MarketScape across both current capabilities and market-readiness of future strategies.
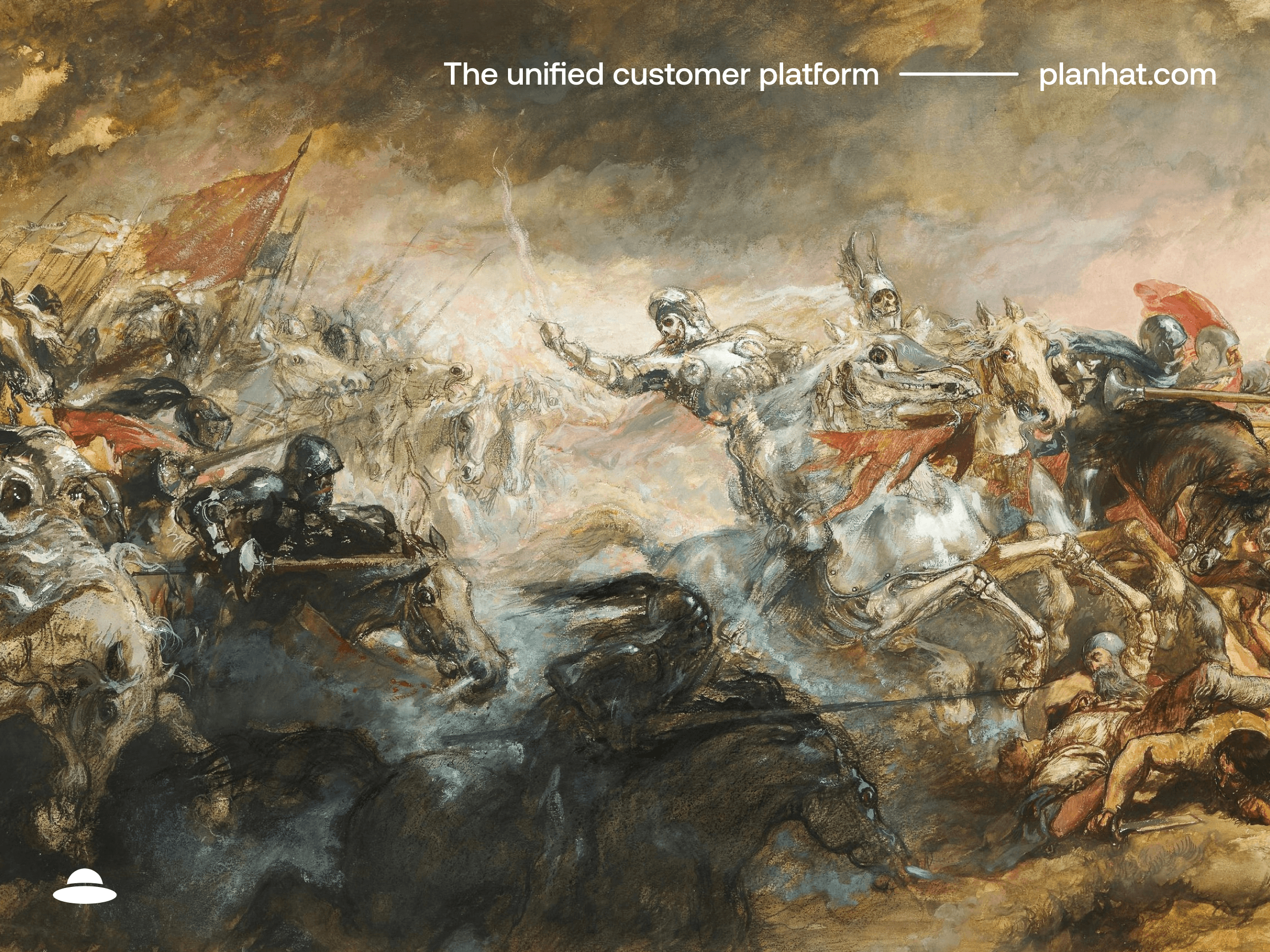
Spotlight
Perspective
•
5 minute read
The infinite frontier
Discover Planhat's philosophy on Security, centered around dynamic protocols rather than static protections.
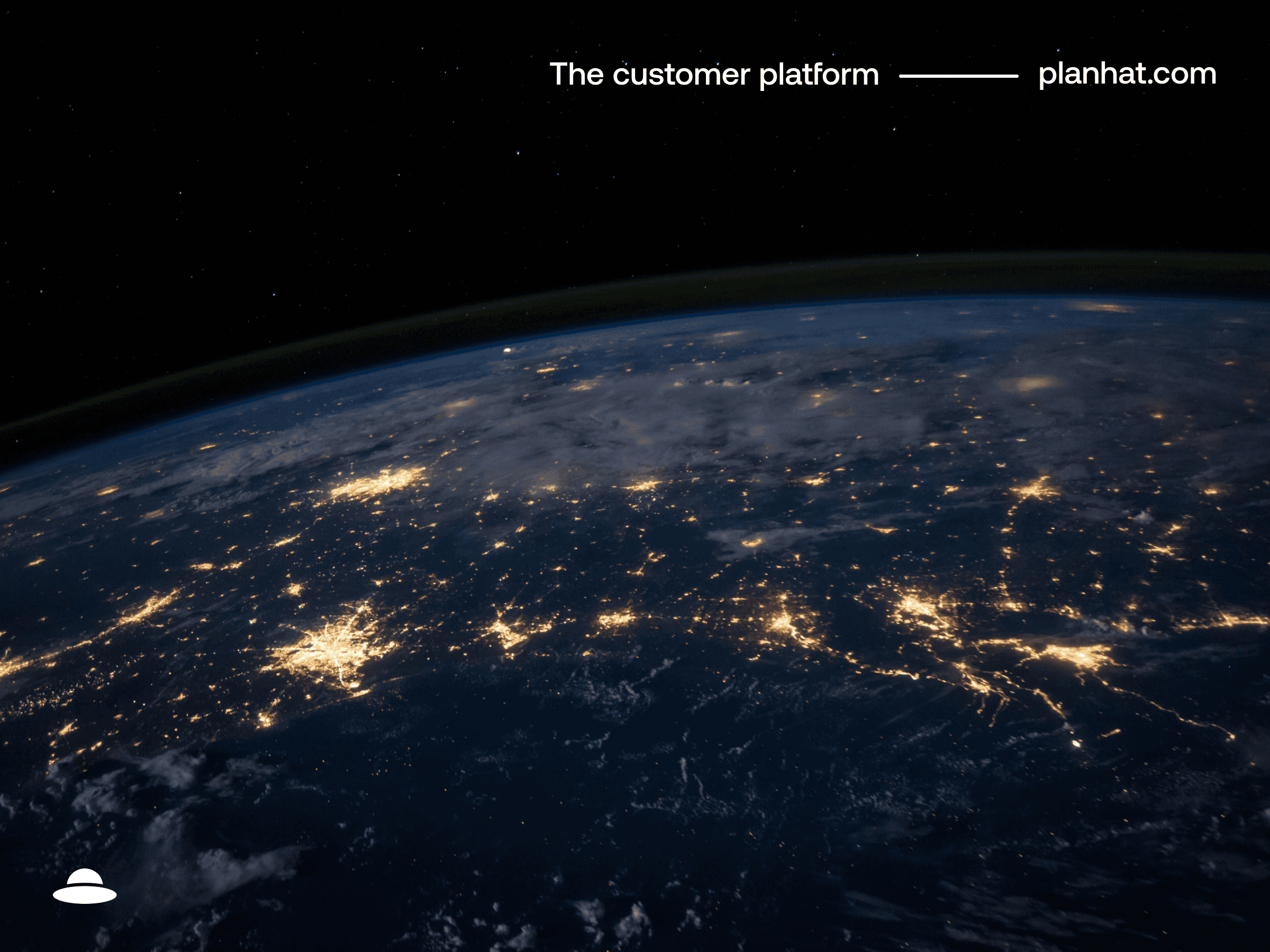
Spotlight
Vendor Assessment
•
10 minute read
Planhat is named a Customer Success Leader by IDC
Planhat is a Leader in IDC's 2024 MarketScape across both current capabilities and market-readiness of future strategies.
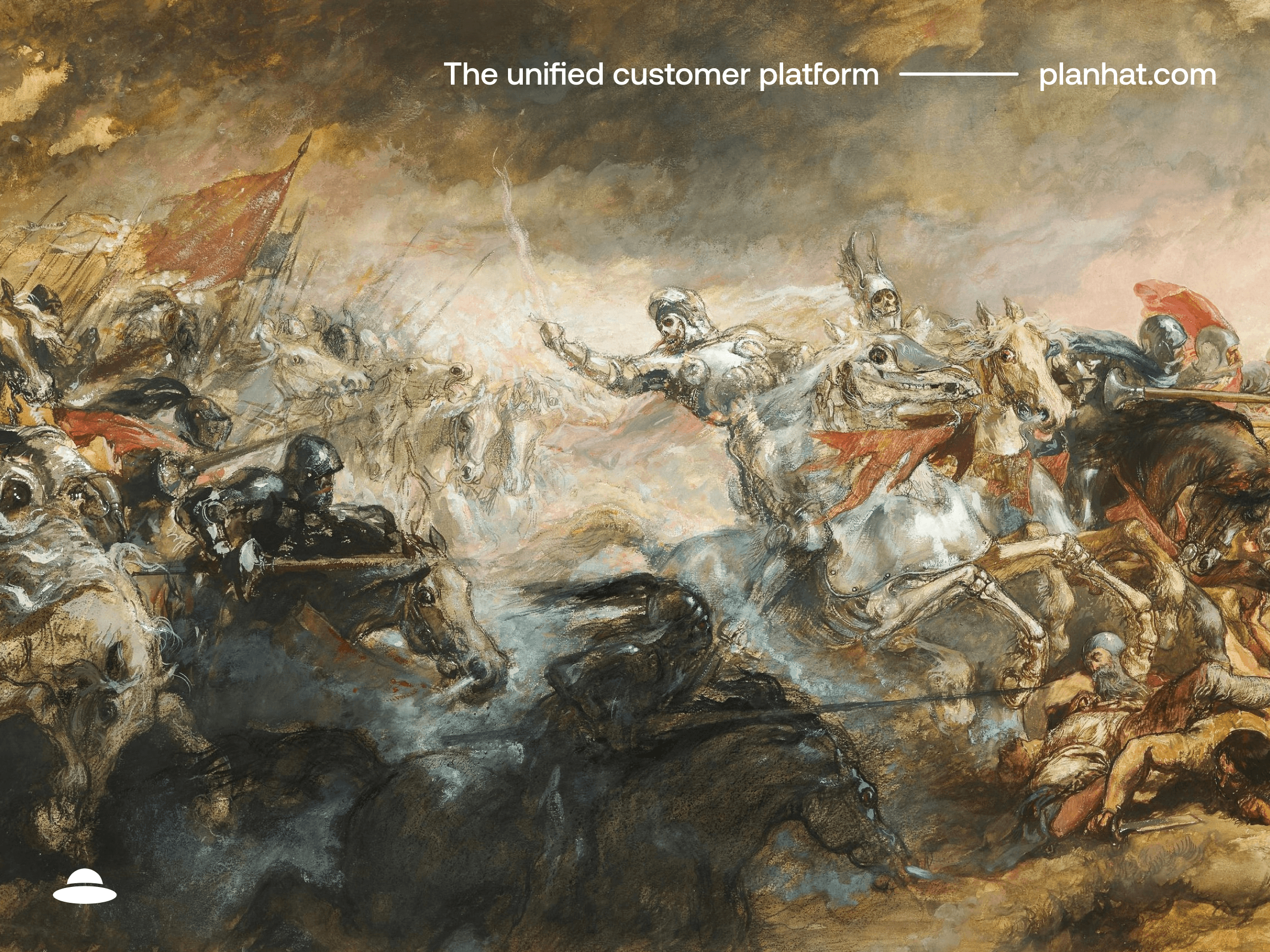
Spotlight
Perspective
•
5 minute read
The infinite frontier
Discover Planhat's philosophy on Security, centered around dynamic protocols rather than static protections.
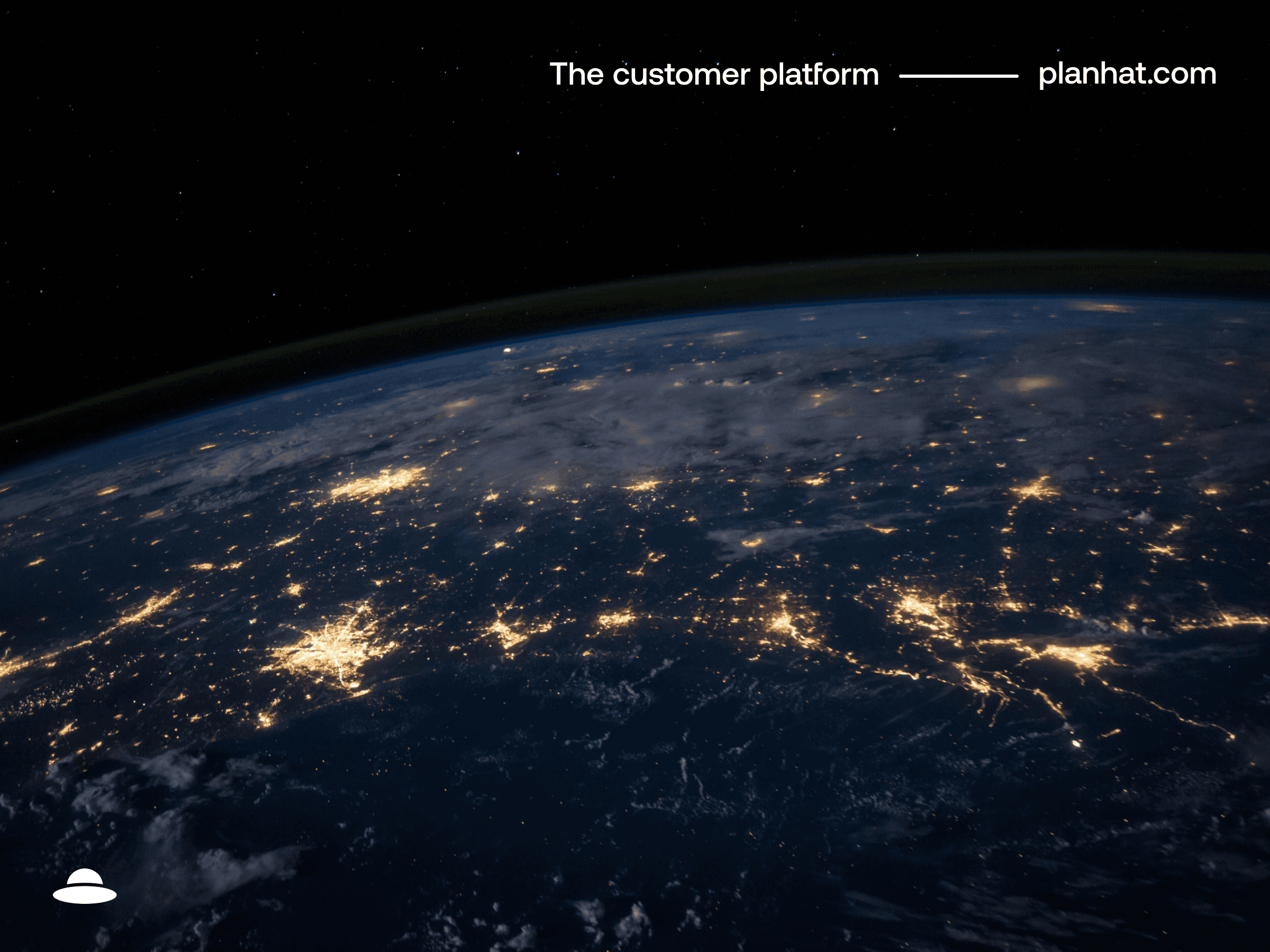
Spotlight
Vendor Assessment
•
10 minute read
Planhat is named a Customer Success Leader by IDC
Planhat is a Leader in IDC's 2024 MarketScape across both current capabilities and market-readiness of future strategies.
Load More
Load More
Load More
Understand Your Customers
Understand Your Customers
Understand Your Customers
Understand Your Customers
Load More
Load More
Accelerate time to value
Accelerate time to value
Accelerate time to value
Accelerate time to value
Load More
Load More
Deliver a world-class experience
Deliver a world-class experience
Deliver a world-class experience
Deliver a world-class experience
Become customer-centric
Become customer-centric
Become customer-centric
Become customer-centric
Load More
Load More
Optimize your customer organization
Optimize your customer organization
Optimize your customer organization
Optimize your customer organization
Load More
Load More
Retain & grow your revenue
Retain & grow your revenue
Retain & grow your revenue
Retain & grow your revenue
Load More
Load More
Our Perspective
Our Perspective
Our Perspective
Our Perspective
Resources
Customers
© 2025 Planhat AB
Resources
Customers
© 2025 Planhat AB
Resources
Customers
© 2025 Planhat AB